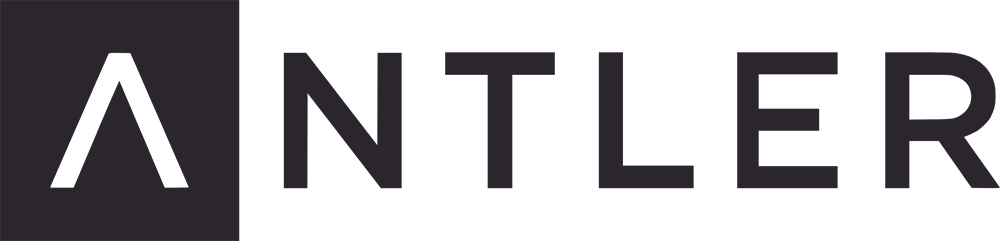

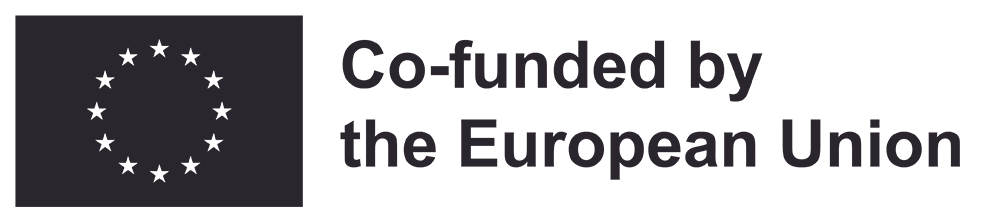


Social-Aware AI
Our multimodal AI system processes the complexity of human behavior in real-time - analyzing body language, facial expressions, voice patterns, and contextual signals simultaneously.
By combining emotional state detection with behavioral analysis, we enable AI that truly understands human interaction.
Decoding human behaviour
Our social intelligence layer integrates with any AI system, enabling it to detect and respond to the non-verbal cues that make up 93% of human communication. By recognizing subtle facial expressions, body language, and voice patterns, your AI can adapt in real-time to users' emotional states and engagement levels.
Our Mission
We believe AI should understand people, not just their words. Our mission is to humanize artificial intelligence by building the social intelligence layer that bridges the gap between AI systems and human interaction.
By teaching machines to recognize and respond to the subtle signals that make communication truly human, we're creating a future where technology adapts to people—not the other way around.
Emotional Intelligence
Our AI simultaneously processes body language, facial expressions and voice patterns, detecting 7 distinct emotional states and emotional intensity.
Real-time Multimodal Analysis
Real-time processing of behavioral, emotional, and contextual data, creating a rich understanding of human interaction that goes beyond words.
Behavioral Analysis
Our system analyzes affect patterns, activity states, and contextual markers to detect subtle behavioral signals and interaction dynamics.
Adaptive Response System
LLM-powered system adapts in real-time to emotional states and behavioral cues, delivering natural conversation flow with context-appropriate tone.
Our Research
Team
Our founding team combines deep AI research, data science, business leadership, and advanced machine learning.
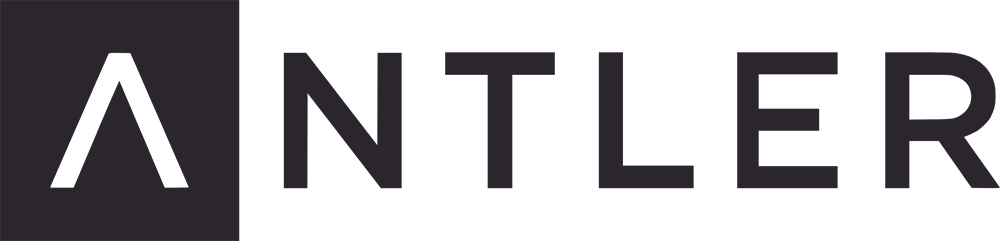

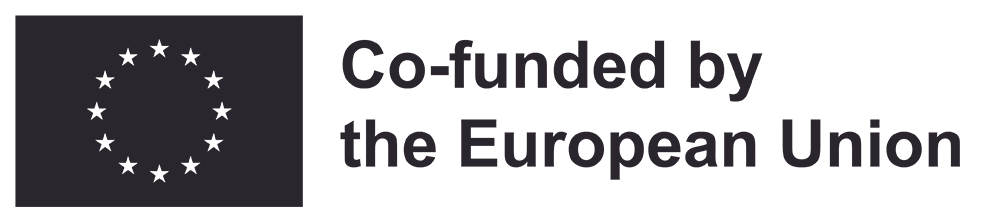

